Archive for year: 2022
Let’s go! Collect Flora Incognita badges for a diverse range of plant observations!
/in Flora Incognita ++, Flora Incognita App/by Anke BebberSince 2018, nature lovers have been identifying and collecting wild flowering plants with our Flora Incognita app, and with the accompanied plant fact sheets, improved their species knowledge constantly. But, as you might know, Flora Incognita is more: Every confirmed observation (the green checkmark in the upper right corner after an identification) contributes to a vast, global collection of plant species observations with which our scientists research changes in biodiversity.
With our latest app release, we want to take this to a new level: We introduce the Flora Incognita badges! Each badge rewards the user for completing certain tasks: Collecting early-blooming flowers or grasses, the tree of the year, ferns or just using the app a couple of days in a row. You will find many challenges to solve – maybe you already have reached first goals?
You will find your collected badges in the profile section of the app. A tap on “Achievements” will lead you to an overview of all the tasks we have prepared for you. We hope this new feature will motivate you to look beyond the usual, take the extra path, examine plants even more closely. We’re looking forward to your feedback! A rating in the app store, a share on Twitter, Facebook or Instagram, or even a recommendation to a friend are ways to spread the news that we highly appreciate!
If you find anything peculiar or working unexpectedly, send us an e-mail. And now, check out if you’ve already earned some badges!
“The advancement of AI methods will create key momentum for environmental and biodiversity research”
/in Project/by floraincognitaInterview with Prof. Patrick Mäder about the interaction of computer science, biology and Big Data
As a young research group at TU Ilmenau, the Data-intensive Systems and Visualization Group (dAI.SY), with currently more than 20 scientists and many student collaborators led by Prof. Patrick Mäder, combines diverse expertise in computer science, engineering and related scientific fields. In addition to machine learning and reliable software, biodiversity informatics has emerged as a focus of the department in recent years. In this way, the research team aims to contribute to the preservation of biodiversity. UNIonline spoke with Prof. Mäder about research in this area.
With your research focus on biodiversity informatics, you are working at the interface of computer science, biology and big data, thus bringing together the topics of digitization and sustainability. What motivated you to conduct research in this area and specifically on the topic of biodiversity?
In 2006 and 2012, I participated in expeditions to Siberia lasting several weeks under the leadership of Prof. Christian Wirth, the founding director of the German Center for Integrative Biodiversity Research iDiv, and Prof. Ernst-Detlef Schulze, founding director of the Max Planck Institute for Biogeochemistry. Together we conducted ecological research under adventurous conditions that was very memorable and changed my awareness of biodiversity: Climate change is a major threat to humanity, and biodiversity loss goes hand in hand with it. We need to stop thinking in silos and bring together the expertise of different scientific fields to study these complex relationships, understand them, and develop foundations for solutions. Our Flora Incognita project is doing just that.
Until recently, biologists did not have access to very large amounts of data to analyze. However, this has changed in recent decades, allowing researchers like you to use these data to investigate ecological questions. What kind of data are you working with?
We work with very different types of data, primarily photos, but also location data, multispectral image data that capture more than the three color channels perceivable by humans and thus information not visible to the human eye, and point clouds , which arecollections of very many measurement points generatedby laser scanners. Probably best known are the observation data that we have been using for years for automatic plant identification in the Flora-Incognita app: Millions of users worldwide ensure every day that we can link image evidence of plants with their locations, enabling us to analyze and predict the distribution of species.
This information is supplemented by curated observations from selected experts via the Flora Capture app. But our research group is not limited to plants. We process microscopic image data for the detection of phytoplankton and insects. In addition, we use special multispectral image data for the automatic identification of pollen. And for evaluating forest stands, we use point clouds generated with LIDAR sensors, a method related to the Radar related method for optical distance and speed measurementWith such data, we can then, for example, make statements about the water quality or help make urban areas more bee-friendly.
As part of the interdisciplinary research group KI4Biodiv – Artificial Intelligence in Biodiversity Research, you would like to work with the Max Planck Institute for Biogeochemistry to further develop and improve these AI methods and technologies in order to monitor biodiversity in different habitats and landscapes efficiently, quickly and automatically. To what extent is biodiversity monitoring a particular challenge for you as a researcher?
To understand the challenges, we first need to look at what biodiversity monitoring means in the first place: it allows us to perceive and document changes in the spatiotemporal occurrence of species. This includes, of course, the distribution of species: Where is diversity declining, where is it increasing? But biodiversity monitoring also includes other things, such as phenology: when do plants bloom, when do they bear fruit, and when do autumn leaves turn colorful? Such monitoring data indicate changes in biodiversity, they are used to investigate the causes of these changes, and they indicate whether strategies and measures to protect biodiversity are working.
Of course, such monitoring also poses major challenges, especially in three areas: it is expensive, requires a lot of time, and requires excellent taxonomic knowledge. Thus, numerous methods and concepts are needed to conduct effective biodiversity monitoring and to overcome the above-mentioned challenges – which is why automated recording and evaluation methods are the focus of research.
Foto: TU Ilmenau/ari
Interview: Technische Universität Ilmenau
Flora Incognita Update
/in Flora Incognita ++, Flora Incognita App/by floraincognitaOur new release brings you a modern and simplified user experience, and a whole bunch of new features to help you further enjoy your plant findings:
Identify Plants:
- The new user interface is more modern and clear.
- It’s now easier to capture and identify a plant in nature.
- You can disable the autofocus with a new camera feature to make it much easier to take sharp photos of small objects.
Collect plant findings:
- You can add pictures to an observation after the observation.
- You can use your own keywords to sort and filter your plant findings more easily.
- You can now view and filter your plant findings on a map.
- You can more easily browse the general species list by using numerous filters.
Availability:
- Even without a personal profile, you can transfer your data to a new device.
- You can save Flora Incognita pictures in your gallery.
Fact sheets:
- We have added extensive information on invasive species in Central Europe.
Deep Learning in Plant Phenological Research: A Systematic Literature Review
/in Flora Incognita ++, Science/by floraincognitaNegin Katal conducted a systematic literature review to analyze all primary studies on deep learning approaches in plant phenology research. The paper presents major findings from selected 24 peer-reviewed studies published in the last five years (2016–2021).
Research on plant phenology has become increasingly important because seasonal and interannual climatic variations strongly influence the timing of periodic events in plants. One of the most significant challenges is developing tools to analyze enormous amounts of data efficiently. Deep Neural Networks leverages image processing to understand patterns or periodic events in vegetation that support scientific research.
“[…]deep learning is primarily intended to simplify the very time-consuming and cost-intensive direct phenological measurements so far.”
Technological breakthroughs powered deep learning approaches for plant phenology within the past five years. Our recently published paper describes the applied methods categorized according to the studied phenological stages, vegetation type, spatial scale, data acquisition. It also identifies and discusses research trends and highlights promising future directions. It is freely available here: https://www.frontiersin.org/articles/10.3389/fpls.2022.805738/full
To understand how scientific research on phenology is done, the different methods to retrieve phenological data need to be clear:
- Individual based observations are for example human observations of plants, cameras installed below the canopy or even reviewing pressed, preserved plant specimens collected in herbaria over centuries and around the globe.
- Near-surface measurements cover research plots on regional, continental, and global scales and are done for example via PhenoCams, near-surface digital cameras located at positions just above the canopy, or drones.
- Satellite remote sensing is done via satelite indices, such as spectral vegetation indices (VIs), enhanced vegetation index (EVI), and more.
Overview of methods monitoring phenology.
Key findings:
The reviewed studies were conducted in eleven different countries (three in Europe; nine in North America, two in South America, six in Asian countries, and four in Australia and New Zealand) and across different vegetation types, i.e., grassland, forest, shrubland, and agricultural land. The vast majority of the primary studies examine phenological stages on single individuals. Ten studies explored phenology on a regional level. No single study operates on a global level. Therefore, deep learning is primarily intended to simplify the very time-consuming and cost-intensive direct phenological measurements so far.
In general, the main phenological stages are the breaking of leaf buds or initial growth, expansion of leaves or SOS, the appearance of flowers, appearance of fruits, senescence (coloring), leaf abscission, or EOS. More than half of the studies focused either on the expansion of leaves (SOS) or on the flowering time.
Across the primary studies, different methods were used to acquire training material for deep learning approaches. Twelve studies used images from digital repeat photography and analyzed those with deep learning methods. The publication shares in-depth information of the different types of digital photography suitable to provide those training data.
Furthermore, it categorizes, compares, and discusses deep learning methods applied in phenological monitoring. Classification and segmentation methods were found to be most frequently applied to all types of studies, and proved to be very beneficial, mostly because they can eliminate (or support) tedious and error-prone manual tasks.
Future trends in phenology research with the use of deep learning
Machine learning methods need huge amounts of data to be trained. Therefore, increasing the absolute number of collected data is one of the key challenges – especially in regions or countries that lack traditional phenological observing networks so far. The paper describes methods and tools that will become important levers to support this kind of research, for example:
- Installing cameras below the canopy that automatically take pictures and submit them over long periods of time are one way to tackle that.
- PhenoCams prove to be a new and resourceful way to fuel further research: With indirect methods that track changes in images by deriving handcrafted features such as green or red chromatic coordinates from PhenoCam images and then applying algorithms to derive the timing of phenological events, such as SOS and EOS. We expect many more studies to appear in the future evaluating PhenoCam images beyond the vegetation color indices calculated so far.
- Citizen Science data from plant identification apps such as Flora Incognita prove to be a long-term source of vegetational data. These images have a timestamp and location information and can thus provide important information about, e.g., flowering periods, similar to herbarium material.
We see that the study of phenology can easily and successfully exploit deep learning methods to speed up traditional gathering and evaluation of information. We, as a research team, are very proud to be a part of that and invite you to play a vital role – in using Flora Incognita to observe the diversity and change of biodiversity around you.
If you have any questions to us regarding our research, don’t hesitate to reach out! You can find Negin Katal on Researchgate and Twitter (@katalnegin), for example.
Publication:
Katal, N., Rzanny, M., Mäder, P., & Wäldchen, J. (2022). Deep learning in plant phenological research: A systematic literature review. Frontiers in Plant Science, 13. https://doi.org/10.3389/fpls.2022.805738
Can grasses be identified automatically via smartphone images?
/in Flora Incognita ++, Science/by floraincognitaCouch grass or ryegrass? Grasses are considered species, that are difficult to identify. The couch grass (Agropyron repens) is feared and often controlled and in gardens. Ryegrass (Lolium perenne), on the other hand, is the basis of many lawn seed mixtures and is a valuable forage grass. The question is: Can you even differentiate them? In our recently published paper, we investigated whether grasses can be automatically recognized and distinguished despite their great similarity. We wanted to know which perspectives are suitable and whether this would even possible without flowers present.
We studied 31 species using images of the inflorescence, the leaves and the ligule. We found that a combination of different perspectives improves the results. The inflorescence provided the most information. If no flowers are present, pictures of the ligule from the direction of the leaf are best suited to distinguish the grass species. All images were taken with different smartphones.
What do we learn from this experiment for our plant identification app Flora Incognita? Even more difficult groups can be well identified automatically, provided suitable images of the correct plant parts are provieded. These newly gained insights will be incorporated into the further development of our app.
In our experiment, we achieved > 96% accuracy for the 31 species when combining all perspectives. We also gained many, many training images that will significantly improve the reliability of the Flora Incognita app for grasses in the future.
Publication:
Rzanny M, Wittich HC, Mäder P, Deggelmann A, Boho D & Wäldchen J (2022) Image-Based Automated Recognition of 31 Poaceae Species: The Most Relevant Perspectives. Front. Plant Sci. 12:804140. https://doi.org/10.3389/fpls.2021.804140
Flora Incognita demonstrates high accuracy in Northern Europe
/in Flora Incognita ++, Flora Incognita App, Science/by floraincognitaThe Flora Incognita project aims to inspire people – to get to know their (botanical) surroundings better, but also to think outside the box and reflect on the possibilities of artificial intelligence, deep learning or biodiversity. A great example of this is what Jaak Pärtel did: As a student project, he investigated the accuracy of the Flora Incognita application in Estonia, Northern Europe and even published a paper about it! With this exceptional project, Jaak won the Estonian National Youth Science Competition! Congratulations, Jaak! Here is a short interview, to share more details.
Hello, Jaak, congratulations on your first place in the Estonian National Youth Science Competition. Would you have imagined that happening when you started the project?
I honestly had no idea about what my project would become in a year. However, I was certain from the beginning that I want to do something that would not be “just another student project”. I got very positive reviews for the project in school, so I thought I would give it a try in the national competition. I was really surprised when I heard the results for the competition. Even that was not all, as I have published a scientific article (co-authors Jana Wäldchen and Meelis Pärtel) based on the project’s dataset and will represent my country in the European Union Contest for Young Scientists 2020/2021 this September.
How did you get the idea to do this project?
My two interests were life sciences and technology, so I found a suitable combination of the two. I had heard of plant identification apps but was not sure how I wanted to have a field works experience and collect an extensive dataset to analyse it statistically.
Can you explain briefly what you investigated and how you went about it?
I investigated the accuracy of the plant identification applications Flora Incognita. I conducted the study in two parts: one with 1500 plant images from a database and second with 1000 observations in Estonian wilderness. I also investigated whether plants with flowers were identified more accurately and how much time automated identification took compared to traditional methods.
What are your main results in the project?
The main result of my project was that both applications reached close to 80% in accuracy in Estonian field conditions, with the correct species among the top five suggestions in circa 90% of the observations. In field conditions, plants with flowers were identified considerably more accurately than ones without them. Automatic identification took one minute compared to over four minutes for manual identification. During my project, I also translated Flora Incognita into my national language – Estonian.
What were you telling bypassers when they saw you documenting flowers? :-)
I had no such situations, as most of my field works took place in locations with little populace. However, I would have said that I am a researcher collecting a dataset about plant apps. A surprising number of people have at least heard about the apps and would probably understand my mission in the field.
What are your next plans?
As of now I am serving my country in mandatory conscription service but after that I will start my Bachelor’s studies of Biology and nature conservation in University of Tartu. I would like to pursue science as a carreer and use innovative and computational methods in biology.
Publication:
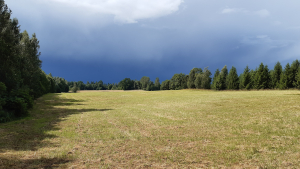
Latest News
Project management
Contact
support@floraincognita.com